Design Principles¶
Distributions are the fundamental building blocks of a POMDP.
Essentially, a POMDP describes the interaction between an agent and the environment. The interaction is formally encapsulated by a few important generative probability distributions. The core of pomdp_py is built around this understanding. The interfaces in
pomdp_py.framework.basics
convey this idea. Using distributions as the building block avoids the requirement of explicitly enumerating over \(S,A,O\).
–
POMDP = agent + environment
Like above, the gist of a POMDP is captured by the generative probability distributions including the
TransitionModel
,ObservationModel
,RewardModel
. Because, generally, \(T, R, O\) may be different for the agent versus the environment (to support learning), it does not make much sense to have the POMDP class to hold this information; instead, Agent should have its own \(T, R, O, \pi\) and the Environment should have its own \(T, R\). The job of a POMDP is only to verify whether a given state, action, or observation are valid. SeeAgent
andEnvironment
.
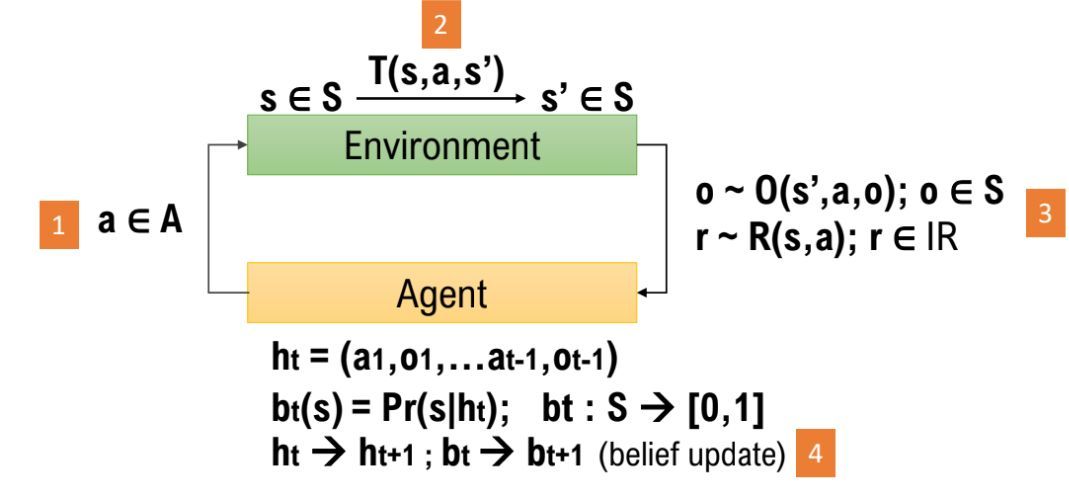
A Diagram for POMDP \(\langle S,A,\Omega,T,O,R \rangle\). (correction: change \(o\in S\) to \(o\in\Omega\))¶